
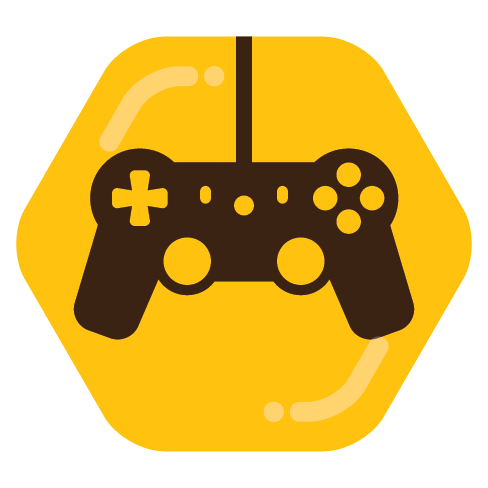
I’d honestly go one step further and say that the problem cannot be fully solved period.
There are limited uses for voice cloning: commercial (voice acting), malicious (impersonation), accessibility (TTS readers), and entertainment (porn, non-commercial voice acting, etc.).
Out of all of these only commercial uses can really be regulated away as corporations tend to be risk averse. Accessibility use is mostly not an issue since it usually doesn’t matter whose voice is being used as long as it’s clear and understandable. Then there’s entertainment. This one is both the most visible and arguably the least likely to disappear. Long story short, convincing enough voice cloning is easy - there are cutting-edge projects for it on github, written by a single person and trained on a single PC, capable of being run locally on average hardware. People are going to keep using it just like they were using photoshop to swap faces and manual audio editing software to mimic voices in the past. We’re probably better off just accepting that this usage is here to stay.
And lastly, malicious usage - in courts, in scam calls, in defamation campaigns, etc. There’s strong incentive for malicious actors to develop and improve these technologies. We should absolutely try to find a way to limit its usage, but this will be eternal cat and mouse game. Our best bet is to minimize how much we trust voice recordings as a society and, for legal stuff, developing some kind of cryptographic signature that would confirm whether or not the recording was taken using a certified device - these are bound to be tampered with, especially in high profile cases, but should hopefully somewhat limit the damage.
The author’s suggesting that smart people are more likely to fall for cons that they try to dissect but can’t find the specific method being used, supposedly because they consider themselves to be infallible.
I disagree with this take. I don’t see how that thought process is exclusive to people who are or consider themselves to be smart. I think the author is tying himself into a knot to state that smart people are actually the dumb ones, likely in preparation to drop an opinion that most experts in the field will disagree with.